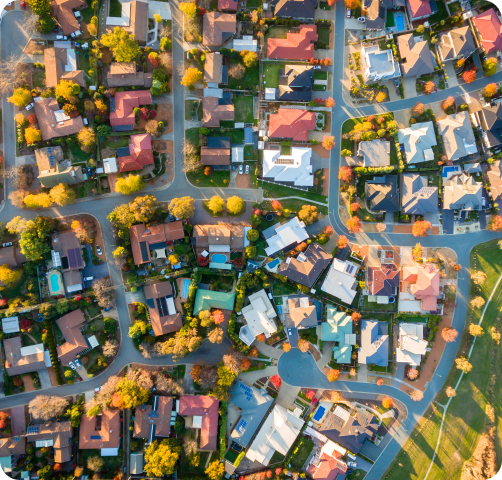
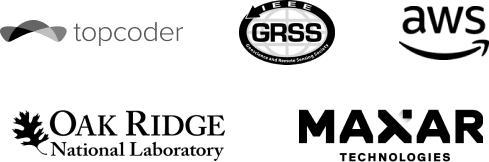
SpaceNet is an initiative dedicated to accelerating open-source, artificial intelligence applied research for geospatial applications, specifically foundational
mapping (i.e., building footprint and road network detection). SpaceNet is run by co-founder Maxar and partners Amazon Web Services (AWS), Topcoder, Oak Ridge National Laboratory and IEEE GRSS.
The Challenge
Challenge Overview
Each year, natural disasters such as hurricanes, tornadoes, earthquakes and floods significantly damage infrastructure and result in loss of life, property and billions of dollars. As these events become more frequent and severe, there is an increasing need to rapidly develop maps and analyze the scale of destruction to better direct resources and first responders.
To help address this need, the SpaceNet 8 Flood Detection Challenge will focus on infrastructure and flood mapping related to hurricanes and heavy rains that cause route obstructions and significant damage. The goal of SpaceNet 8 is to leverage the existing repository of datasets and algorithms from SpaceNet Challenges 1-7 and apply them to a real-world disaster response scenario, expanding to multiclass feature extraction and characterization.
Since its launch in 2016, SpaceNet has made significant progress advancing open-source building footprint and road extraction algorithms. During SpaceNet 8, challenge participants will train algorithms on imagery and labels from previous challenges—as well as newly created labeled training datasets from Maxar—to rapidly map an area affected by flooding. Any winning open-source algorithm from SpaceNet 1-7 may also be used.
SpaceNet 8 aims to answer these questions:
- How have algorithms that extract buildings and roads improved since SpaceNet was launched, and how can top algorithms from previous challenges be leveraged?
- What is the impact on performance for a multiclass feature extraction challenge—i.e., buildings and roads?
- How accurately can roads obstructed by flood waters be characterized from pre-event road detections and post-event satellite imagery?
Why Foundational Mapping Matters
The commercialization of the geospatial industry has led to an explosive amount of data being collected to characterize our changing planet. One area for innovation is the application of computer vision and deep learning to automatically extract information from satellite imagery at scale. Today, map features such as roads, building footprints, and points of interest are primarily created through manual techniques. The SpaceNet team believes that advancing automated feature extraction techniques will serve important downstream uses of map data such as humanitarian and disaster response. Further, solving foundational mapping challenges is an important step to unleash the power of advanced computer vision algorithms applied to a variety of remote sensing applications.
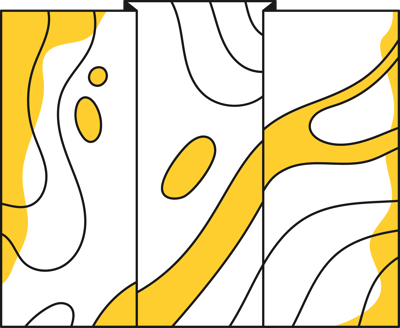
$50,000
in total challenge prizes
New to Topcoder?
Join the Topcoder Community so you can participate in this amazing challenge and many more like it!
